The Science Behind AI Content Detectors: Insights into Their Methods and Accuracy
Introduction to AI Content Detectors
In the digital age, where content creation and consumption occur at an unprecedented scale, the ability to distinguish between human-generated and machine-generated text is becoming increasingly important. AI content detectors are at the forefront of this technological frontier, offering a means to automatically identify AI-generated content for various applications, from academic honesty to the integrity of online reviews.
At the core of these detectors lie sophisticated concepts from the fields of computer science and linguistics. Understanding these underlying principles is essential for grasping how AI content detectors function and their significance in our society. So, what are the key concepts such as machine learning, deep learning, and automated content detection that power these tools?
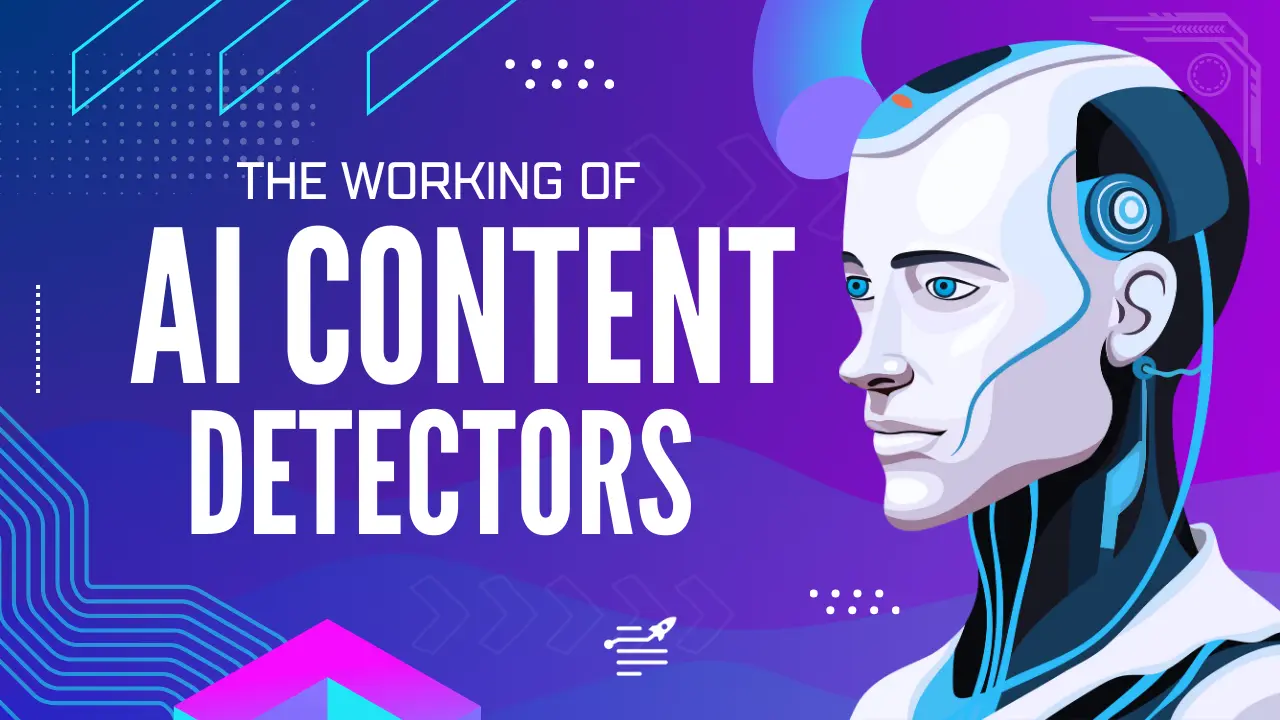
Machine Learning and Deep Learning
Machine learning is a subset of artificial intelligence that involves training algorithms to make predictions or decisions without being explicitly programmed to perform the task. These algorithms improve their performance as they process more data. Deep learning, a more advanced subset of machine learning, utilizes neural networks with multiple layers to analyze vast amounts of data, learn from it, and make complex decisions.
Both machine learning and deep learning are instrumental in automated content detection. By analyzing patterns in language use, syntax, and structure, these technologies enable AI content detectors to differentiate between content created by humans and machines.
Automated Content Detection
Automated content detection refers to the use of algorithms to identify specific characteristics within text data. These characteristics may include stylistic elements, grammar patterns, and other linguistic features. The goal is to flag content that deviates from normative human writing—an indication that it might be AI-generated.
The relevance of AI content detectors spans several sectors. For educators, they can serve as tools to ensure academic integrity by detecting potential cases of plagiarism or outsourcing assignments to AI writing services. In e-commerce, they help maintain the authenticity of product reviews by screening out AI-generated fake reviews that could mislead consumers. Moreover, they play a role in preserving the quality and credibility of journalistic sources, online forums, and social media platforms.
Now that we've laid the groundwork by introducing the critical concepts behind AI content detectors, we're ready to delve deeper into the methods they use and their accuracy rates. These methods are not just about raw computational power but also about the subtleties of human language and how AI interprets and mimics it. As we will see, while AI content detectors leverage cutting-edge technology, they are still bound by the complexities and nuances of language—a field where even humans can struggle to discern intent and authenticity.
Fundamentals of AI Detection Methods
Peeling back the layers of AI content detectors reveals a world where nuances in language are scrutinized. But what specific features are these detectors looking for? Let's dive into the stylometric traits they analyze to tell apart the writings of algorithms from those of humans.
Stylometric Features: Vocabulary and Beyond
Imagine a detective combing through a manuscript, searching for clues to the writer's identity. This is akin to how AI detectors scrutinize text, but instead of a magnifying glass, they use stylometric features. Vocabulary richness, or the diversity of words used by a writer, can be a telltale sign. Too homogeneous, and it might hint at an AI's handiwork. Sentence length and complexity also play roles; AI tends to favor more uniform structures, whereas human writing exhibits greater variation. Punctuation usage—the sprinkling of commas, periods, and semicolons—can have its own rhythm and flow that AI might not fully replicate. And then there's readability: text that flows too perfectly, without the occasional stumble of complex syntax or rare vocabulary, might just be too good to be true, indicating an AI's involvement.
Classifiers: The Decision-Makers
Within the core of an AI detector lies the classifier—a decision-making entity trained to spot differences between AI-generated and human-written text by analyzing the aforementioned stylometric features. By feeding it countless examples of both types of writing, the classifier learns to pick up on subtle patterns. For instance, does the piece include idiosyncratic expressions or is the diction unusually standardized? These classifiers act as gatekeepers, constantly refining their criteria to ensure accuracy in their verdicts.
Algorithms: The Underlying Mechanics
Beyond mere feature spotting, AI content detectors deploy sophisticated algorithms to make sense of the data. N-grams, sequences of 'n' items from a given sample of text, help in understanding context and predicting the likelihood of certain word combinations appearing in human vs. AI-written text. Syntax analysis delves deeper into the arrangement of words and phrases to create well-formed sentences, while lexical analysis breaks down the text into its lexical components, such as tokens and symbols, to assess whether the writing style corresponds more closely to a machine or a human author.
These methods are part of a complex dance between pattern recognition and anomaly detection, where even the slightest misstep can reveal the true authorship of a text. As we move forward in this blog, we'll see how these methods play out in real-world applications and how their accuracy is evaluated.
The Process of AI Content Detection
Delving into the intricacies of AI content detection, let's embark on a step-by-step journey to understand how these sophisticated systems scan and analyze content. Imagine an invisible detective tirelessly sifting through lines of text, seeking the subtle fingerprints left by its digital counterpart.
Step-by-Step Explanation
The initial step involves the input of text by the AI detector. Here, it doesn't simply skim through; it meticulously examines each word and sentence structure, akin to a literary connoisseur. This process includes breaking down the content into quantifiable features such as sentence length, complexity, and vocabulary usage, which we touched upon in the previous section.
Next comes the analysis phase. The AI detector, equipped with machine learning models, starts to search for patterns that are common in AI-generated text. It's like solving a puzzle, where each piece is a stylometric trait, and the finished picture reveals whether a human or a machine wrote the text. The models look for clues such as perplexity, a measure of predictability, and burstiness, which relates to variations in sentence structure and length.
After the analysis, the detector arrives at a decision. It provides a probability score indicating the likelihood of the text being AI-generated. This score is the culmination of intricate calculations and learned patterns, offering a verdict on the text's origin.
Machine Learning's Role
Machine learning models are the core of AI content detectors, acting as the brain that learns from examples. These models are trained using vast amounts of text data from both human and AI writers. Through this training, they develop an ever-improving intuition for distinguishing between the two.
As these models encounter more examples, their accuracy improves, honing their ability to detect even the most nuanced AI-generated texts. They learn not only from the successes but also from the mistakes, adapting their algorithms to better recognize the evolving sophistication of AI writing tools.
Challenges and Limitations
However proficient these detectors may be, they face significant challenges. Complex content, with its intricate language and creative flair, can sometimes resemble the unpredictability of human writing, throwing off even the most advanced detectors.
Moreover, as AI writing tools evolve, they learn to mimic human idiosyncrasies more closely, making the task of detection akin to finding a needle in a haystack. In some cases, AI-generated content might even pass as human-written if it cleverly includes varied sentence structures, colloquialisms, and creative expressions that reduce its perplexity and increase its burstiness.
Another challenge stems from the sheer volume of content needing to be analyzed and the computational power required to process it all. Detectors must be efficient and scalable while maintaining high accuracy—a balancing act of technological prowess.
In light of these obstacles, the question arises: How far can we trust these detectors? While they provide valuable assistance, they are not infallible and should be used as one of several tools in verifying content authenticity.
By understanding the process, the role of machine learning, and the challenges faced, we gain a deeper appreciation for the technological dance between AI content creation and detection. It's a continuous game of cat-and-mouse, with each side evolving to outsmart the other. And as we will explore further, these advancements raise pertinent ethical questions and pave the way for future developments in the field.
Evaluating the Accuracy of AI Detectors
The accuracy of AI content detectors is a pressing topic as the prevalence of AI-generated text grows. Key factors influence these detectors' ability to discern between human and machine-written content. But what are those factors, and how do they affect the detection process?
Factors Influencing AI Detector Accuracy
Several elements play into the reliability of AI detectors. The training data's quality and diversity, for instance, are crucial; a rich dataset ensures the model learns a wide range of writing styles. Algorithms must also adapt to the evolving sophistication of AI writers, which continuously learn from their interactions and improve over time. Another aspect is the context in which the text is used; some genres or topics may have more distinctive patterns that aid detection.
Comparison with Traditional Methods
Traditional methods, such as plagiarism checkers, often rely on direct comparisons with known sources. AI detectors go beyond this by analyzing the style and structure of the text itself. They look for telltale signs that might not be apparent at first glance, such as subtle consistencies in phrasing or the overuse of common syntactic structures that can suggest the absence of human touch.
Case Studies Showcasing Effectiveness
- Various tools like Originality.ai and Copyleaks claim high accuracy, with the former boasting up to 99% accuracy and the latter citing a 0.2% false positive rate. These figures, impressive as they are, should be taken with caution, considering the challenges AI detectors face.
- An article from The Blogsmith highlights the inconsistency in AI detectors' performance. In some cases, human-written content was flagged as AI-generated, leading to potential misunderstandings and trust issues.
- According to a study in the International Journal for Educational Integrity, false positive rates were relatively low across different tools, but the true positive rates varied widely, ranging from 19.8% to 98.4%.
Understanding these dynamics is essential as we navigate the complex dance between AI content generation and its detection. The arms race between creating and detecting AI content continues, with both sides constantly evolving.
Important Note: Google emphasizes content quality over its origin, confirming that AI-generated content is acceptable as long as it delivers value. The key criterion for Google is whether the content is valuable, not whether it's created by humans or AI. Content that fails to provide value is consistently downplayed
Ethical Implications of AI Content Detection
Peeling back the layers on content moderation, we find ourselves at the heart of a pressing issue: the ethical implications of using AI detectors for this purpose. The crux lies not only in deciding what is permissible but also in addressing the inherent moral questions that surface when machines judge the appropriateness of content.
Content Moderation and Ethics: A Delicate Dance
Content moderation, traditionally a human endeavor, has evolved with AI stepping onto the dance floor. As these detectors become more ingrained in online ecosystems, several ethical considerations come to light. They range from the responsibility of ensuring fairness to the challenge of maintaining transparency in automated processes. A paramount concern is bias; an AI's judgment is only as equitable as its programming and data sets allow. Issues of privacy are equally significant, with fears that personal data could be used without consent, breaching individual rights and freedoms.
Tackling Bias and Fairness in AI-Driven Moderation
Bias in AI content detectors is not just a possibility; it's an echo of the data they consume. If the training data skews towards certain demographics or viewpoints, so too will the decisions of the AI and AI hallucinations will be inevitable. This can lead to unfair censorship or promotion of content, which may marginalize voices and perspectives. Addressing this requires a commitment to diversity and balance within the datasets and constant vigilance to evaluate outcomes for signs of partiality. The transparency of these systems is also critical—stakeholders should understand how and why decisions are made.
Privacy Concerns in the Age of AI Surveillance
Privacy concerns loom large as AI detectors require vast amounts of data to function efficiently. The collection and storage of such data present a conundrum: How do we ensure the confidentiality and integrity of user information? This becomes especially tricky when AI models are trained on publicly available content without explicit user consent. It raises questions about the ownership of data and the ethics of its use in content detection systems.
Transparency and Accountability in AI Systems
A lack of transparency in AI operations can sow distrust. Users have the right to understand the mechanics behind content detection and the reasoning for content being flagged or removed. This involves clear communication regarding AI decision-making processes and the ability to appeal or seek clarification on contentious verdicts. Ensuring accountability within these systems necessitates a framework where those deploying AI for content moderation are held responsible for the ethical implications of their use.
Charting the Course: Ethical Guidelines and Best Practices
To navigate the grey waters of ethics in AI content detection, we must chart a course that considers both current challenges and future ramifications. Establishing ethical guidelines is imperative for developers and users alike. These might include stringent data protection measures, transparency logs for AI decisions, and regular audits to check for bias. Encouraging best practices such as integrating human oversight and ensuring diverse representation in training data can also go a long way in mitigating risks.
In the vast ocean of digital content, AI detectors serve as both navigators and gatekeepers. But without a moral compass, these tools could steer us into troubled waters. As we continue to explore the capabilities of artificial intelligence, let us remain steadfast in our commitment to upholding the highest ethical standards. With careful consideration and action, we can ensure that AI content detection not only serves its intended purpose but does so with integrity and respect for the principles we hold dear.
Future Trends in AI Content Detection
As we delve into the future trends of AI content detection, it's crucial to understand how rapidly this technology is advancing. The implications for digital content creation and management are significant, with the potential to reshape the landscape of online information.
Prediction of Future Advancements in AI Content Detection Technology
AI content detectors have already made impressive strides in distinguishing between human-written and AI-generated texts. However, as AI algorithms become more sophisticated, we can anticipate even more intricate methods surfacing as removing AI detection from AI generated content would become increasingly feasible.
One area where significant advancements are expected is in deep learning architectures. These systems will likely evolve to understand context and narrative flow at a much deeper level, making them more adept at identifying subtle nuances in language that may indicate AI authorship.
Moreover, as reinforcement learning continues to mature, AI detectors could refine their accuracy through ongoing feedback loops, essentially learning from their 'mistakes' and improving over time.
Analysis of Potential Applications Beyond Plagiarism Detection
The applications of AI content detectors extend far beyond simply acting as a plagiarism-checker. Content security is one such area, as organizations seek to protect their proprietary and confidential information from unauthorized distribution. AI detectors could play a pivotal role in identifying and flagging sensitive data before it leaves a secure environment.
Another burgeoning application is text analysis for sentiment, tone, and intent, which has profound implications for fields like marketing, political campaigns, and public relations. These tools can analyze vast quantities of text to derive insights that would be impractical for humans to gather on their own.
Consideration of the Evolving Landscape of AI Detectors and Their Impact on Digital Content Creation and Management
The evolution of AI content detectors isn't happening in a vacuum; it's part of a broader shift in the digital content sphere. As these tools become more prevalent, content creators and managers must adapt. The rise of AI-generated content across industries has been both a boon and a challenge, necessitating the need for robust detection tools. This cat-and-mouse game between AI creators and detectors will likely result in a continuous cycle of innovation. Furthermore, as AI becomes more integrated into content creation and management workflows, it will be essential to maintain transparency and ethical standards to foster trust among users and consumers.
Conclusion
The effectiveness of AI content detectors hinges on their ability to discern the subtle nuances that differentiate AI-generated text from human writing. As we've seen, this is no small feat. AI writing tools are becoming increasingly sophisticated, often mimicking the perplexity and burstiness characteristic of human prose. With advancements in machine learning algorithms and deep learning techniques, these detectors have grown more adept at flagging content that veers towards the predictable nature of AI-generated text.
Looking ahead, the horizon of AI content detection is both promising and challenging. We can anticipate further enhancements in detection capabilities, potentially leading to broader applications beyond the realm of plagiarism and spam filtering. The potential incorporation of watermarking systems, as suggested by OpenAI, hints at future methods for ensuring the authenticity of digital content. Yet, the continuous evolution of AI writing tools means that content detectors will need to persistently adapt and improve to stay effective.
As we move forward, it is incumbent upon us to navigate this space thoughtfully, balancing the benefits of AI detection with the values of honesty and trust that underpin our digital interactions.