Are AI Detectors Truly Accurate? Deep Diving into How AI Detectors Work?
Understanding AI Detectors
At the heart of the rapid growth of AI technologies we are left with one critical question: how can we tell if what we're reading was penned by human intellect or synthesized by the clever algorithms of artificial intelligence? AI detectors step into this intriguing arena with a goal—to differentiate between the two. These digital tools analyze text, seeking out the subtle hints that may reveal the nature of its origin. But what are these AI detectors, and why do they matter?

The Role of AI Detectors in Detecting AI-Generated Content
AI detectors are sophisticated tools designed to flag content that is likely generated by AI, such as language models. They operate by analyzing writing patterns, formatting choices, and other textual features that may signify non-human authorship. These detectors provide a probability score indicating the chance that a piece of text was AI-crafted rather than human-written.
The accuracy of these tools, however, can vary widely, influenced by factors like the complexity of the analyzed content and the specific detection tool in use.
Different types of AI Detectors
Not all AI detectors are created equal. Some rely on the analysis of perplexity—the unpredictability or randomness in a piece of text—while others may focus on semantic coherence or the presence of repetitive patterns that tend to be hallmarks of AI-generated content.
There are also more nuanced detectors that delve into linguistic intricacies, attempting to find out the 'fingerprint' left by AI through its training data. The variety of approaches reflects an ongoing arms race: as AI becomes more adept at mimicking human writing, detectors must evolve to maintain their edge.
Application of AI Detectors in Different Fields
The implications of AI detection stretch far beyond mere curiosity. In academia, detectors serve as plagiarism checker, ensuring the integrity of scholarly work. Media platforms leverage them for content moderation, weeding out inauthentic material. The financial sector even employs these tools for fraud prevention, recognizing the potential for AI to craft convincing phishing emails or forge documents.
In every case, the goal is to maintain a level of authenticity and trust that AI-generated content might otherwise compromise.
Important Note: Google emphasizes content quality over its origin, confirming that AI-generated content is acceptable as long as it delivers value. The key criterion for Google is whether the content is valuable, not whether it's created by humans or AI. Content that fails to provide value is consistently downplayed.
Factors Influencing Accuracy of AI Detectors
Training Data Quality and Model Complexity
The foundation of any AI detector lies in its training data—the more comprehensive and high-quality this dataset is, the better the AI can learn to distinguish between human and machine-generated text.
An AI detector trained on a diverse array of writing styles, topics, and nuances will have a more refined ability to analyze new content.
Model complexity also plays a significant role. Simpler models may not capture the subtleties of language as effectively as more complex ones. However, with increased complexity comes the challenge of interpretability—understanding why the model made a particular decision—which is vital for improving and trusting the system.
Challenges in Distinguishing AI from Human Content
AI detectors face a continually evolving challenge: the AI-generated content is becoming increasingly sophisticated. As AI models like ChatGPT advance, the line between human and machine writing blurs, making the detector's job harder. The AI detectors must constantly update to keep pace with the advancements in language models. This arms race can lead to inconsistencies, where newer AI content might slip past older detection algorithms, as evidenced by varying success rates in identifying different versions of ChatGPT-generated text.
Bias
Bias is an insidious factor that can creep into AI detectors, often stemming from the data they were trained on. If the training data set is skewed towards certain types of content or lacks diversity, the AI detector may develop blind spots or a propensity to flag false positives/negatives inaccurately. For example, if an AI detector has been predominantly trained on formal academic writing, it might struggle to accurately assess more casual or creative content.
Context, and Linguistic Nuances
Context and linguistic nuances further complicate matters. A piece of text could be entirely factual but written in a style that mimics AI-generated content, or vice versa. AI detectors have to navigate this minefield of stylistic variations and contextual cues to make accurate judgments.
Case Studies on AI Detector Accuracy
A particular study noted in the International Journal for Educational Integrity presents an insightful evaluation of AI detectors. According to the report, one detection tool had a success rate of 60% in correctly identifying AI-generated text. Though this may seem like a passable performance at first glance, it indicates a considerable margin of error, especially when the origin of the text is unknown. This uncertainty could lead to misclassifications, impacting the credibility of the content and its creators.
Another example comes from GPTRadar, which showed a 64% confidence level in categorizing a sample as AI-generated content. While not the absolute measure of accuracy, this confidence level provides insights into how certain the tool is about its classification, reinforcing that there's room for improvement.
On the other end of the spectrum, the ZeroGPT AI text detector boasts an impressive over 98% accuracy rate. However, such high rates often come with caveats, such as limited testing conditions or selection bias in the training datasets.
Strengths and Limitations in Various Scenarios
The efficacy of different AI detectors can vary significantly depending on the scenario. For instance, the study above found that AI detection tools were more adept at recognizing content generated by GPT 3.5 than the more advanced GPT 4.
Yet, these tools showed inconsistencies and false positives when evaluating human-written content, suggesting a potential for misclassification that could affect users who are wrongly flagged as using AI-generated text.
Performance Across Languages and Styles
The performance of AI detectors is not uniform across various languages, genres, and writing styles. Some tools may excel in English but falter in other languages due to a lack of representative data in their training sets.
The genre and writing style can also present challenges, as creative writing might be harder to distinguish from AI-generated content compared to more formulaic texts. A comprehensive examination is necessary to fully assess the versatility of these detectors
.webp)
Ethical Implications of AI Detector Inaccuracy
Examining Ethical Concerns of Misattribution
Inaccurate AI detectors can inadvertently undermine the integrity of authors and creators. When a human's work is flagged incorrectly as AI-generated, it calls into question their authenticity and effort, potentially causing reputational damage.
Conversely, if an AI's output evades detection and is mistaken for a human's work, it could lead to unfair advantages or plagiarism concerns. This is particularly problematic in academic settings where originality is of prime importance.
Potential Consequences of False Positives and Negatives
The potential for false positives and negatives by AI detectors holds significant ethical implications. A false positive might lead to unwarranted scrutiny or accusations of dishonesty towards a content creator, while a false negative could allow plagiarized or AI-generated content to pass as authentic, compromising academic integrity and intellectual property rights.
These misclassifications don't just affect individuals but can also sway public opinion and erode trust in information authenticity.
Improving the Accuracy of AI Detectors
Addressing Biases and Enhancing Fairness
To mitigate these ethical concerns, it's crucial to address inherent biases within AI detectors. Ensuring a diverse and representative dataset during training can minimize the risk of bias. Additionally, transparency about the limitations and accuracy rates of these tools can help manage expectations and foster an environment where AI detectors are used as one of several tools for verification.
Continuous Learning and Feedback Mechanisms
The dynamic nature of language and content creation necessitates AI detectors that evolve over time. Continuous learning, a process where AI models are perpetually updated with new data, is crucial for maintaining relevancy and accuracy.
Feedback mechanisms, such as user reporting inaccuracies or AI-generated content that slips through the cracks, provide valuable data for refinement. An adaptive AI detector, much like the algorithms it seeks to identify, must learn from its environment to improve its detection capabilities.
Integrating Human Oversight and Interpretability
Human oversight is indispensable for refined decision-making. Incorporating human judgment can help navigate the gray areas where AI may falter, such as understanding sarcasm or literary creativity that may be mistaken for AI-generated text.
Interpretability also plays a key role; if the AI detector's reasoning process is transparent, humans can better understand and trust the outcomes.
Transparency, Accountability, and Validation Processes
For AI detectors to be reliable, transparency in how they operate is essential. If users understand the mechanics behind the detection, they can make informed decisions about the trustworthiness of the results.
Holding AI detectors accountable through rigorous validation processes, such as benchmarking performance across different data sets and scenarios, ensures that claims of high accuracy
Furthermore, validation should be an ongoing process—similar to updating object detection models with new data—to adapt to the evolving landscape of digital content.
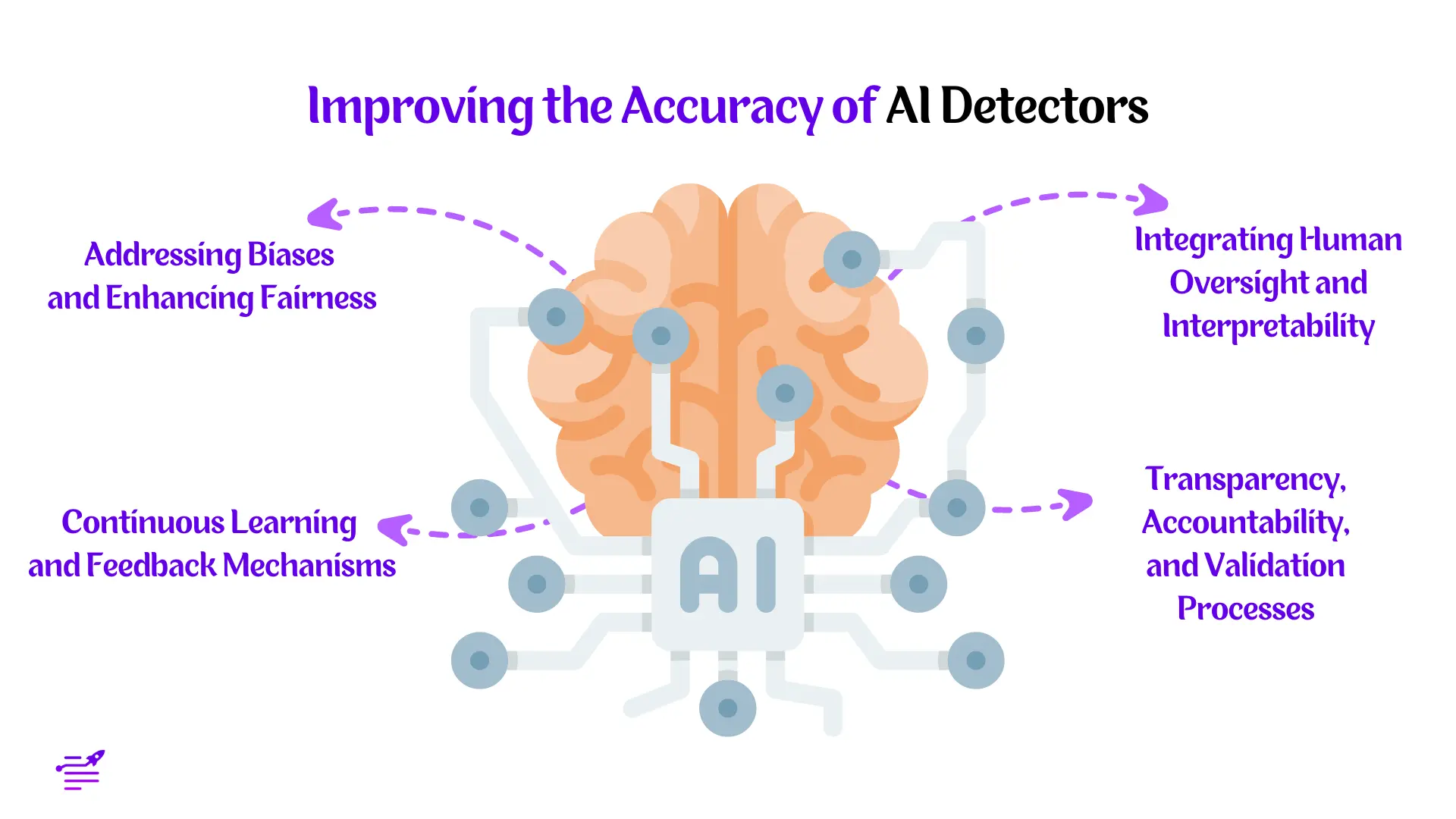
Conclusion and Future Directions
The path forward calls for robust research initiatives focused on enhancing the precision of AI detectors. Considering the dynamic nature of AI-generated content, which is becoming increasingly sophisticated to create personalized content, continuous advancements in detector technology are paramount.
Future research must aim to fine-tune these systems, leveraging larger and more diverse datasets and exploring the integration of advanced machine learning techniques to better capture the subtle differences between human and AI writing styles.
The evolution of AI-generated content also invites us to critically evaluate the outputs of AI detectors. Encouraging discourse among users, developers, and stakeholders can foster a culture of transparency and accountability. By sharing insights and experiences, we can collectively contribute to the refinement of these tools, ensuring that they remain effective against the backdrop of rapidly advancing AI capabilities.
Ultimately, the goal is to ensure that AI detectors not only maintain a high degree of accuracy but also uphold ethical standards. False positives and negatives carry significant implications, especially in sectors where the stakes are high, such as education and security. Therefore, the incorporation of human oversight and interpretability into AI detector systems is essential. It adds a layer of discernment that purely automated processes may overlook, particularly when dealing with nuanced or borderline cases.