Understanding AI Hallucinations
Definition of AI Hallucinations
In the realm of artificial intelligence, a phenomenon known as AI hallucinations occurs when machines generate outputs that deviate from reality. These outputs can present false information or create misleading visuals during real-world data processing. For instance, an AI answering that Leonardo da Vinci painted the Mona Lisa in 1815—centuries after the actual event—is displaying what we term an AI hallucination. The concept is analogous to human hallucinations but manifests uniquely within the digital cognitive processes of AI systems.
Comparison to Human Hallucinations
While AI hallucinations mirror the human experience of perceiving something that isn't there, the underlying causes differ. In humans, these perceptions may be due to chemical reactions or abnormalities in the brain. AI hallucinations, on the other hand, stem from issues such as insufficient training data or model biases. This distinction underscores the difference between organic and artificial cognition, highlighting the mechanical nature of AI errors as opposed to the complex biological processes in humans.
Causes of AI Hallucinations
Overfitting in Training Data
Overfitting is a fundamental cause of AI hallucinations where the model learns patterns that are too specific to the training data, losing the ability to generalize to new data. This leads to high accuracy on training datasets but poor performance on unseen data, often resulting in nonsensical predictions.
Training Data Bias & Factual Inaccuracies
AI models are only as good as the data they are trained on. If the data includes biases or inaccuracies, the AI is likely to replicate these in its outputs, producing hallucinations that reflect flawed information. This is a critical issue, as it can perpetuate existing prejudices or spread falsehoods.
High Model Complexity
Complex models have an increased risk of hallucinating because they might spot spurious correlations in the data. The vast number of parameters in such models can lead to interpretations that are abstract and disconnected from human logic, which might not align with reality or common sense.
Fabricated Information
Another source of AI hallucinations can be entirely fabricated information that the model generates. This may happen when the AI combines elements from various inputs in novel but incorrect ways, leading to plausible-sounding but ultimately false statements or visual misrepresentations.
Examples of AI Hallucinations
ChatGPT Example
ChatGPT, a prominent artificial intelligence language model, sometimes generates outputs that deviate from factual accuracy, referred to as "hallucinations." These inaccuracies can potentially misguide users, prompting questions about the overall efficacy and dependability of AI models.
We will delve into concrete instances of such hallucinations, tracing ChatGPT's evolution and its foundational concepts.
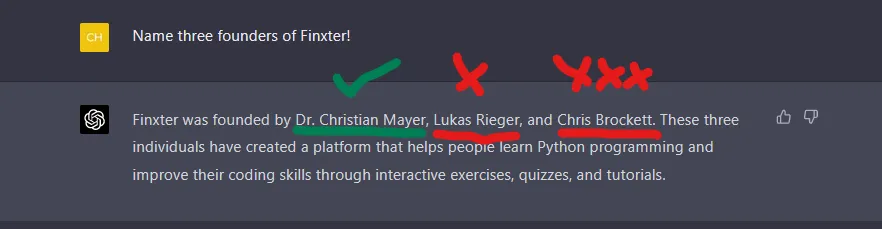
Image Recognition Example
AI hallucinations also occur in image recognition. A famous case involved Google’s Photos app mistakenly labeling African Americans as gorillas, a result of training data deficiencies and algorithmic shortcomings. Such errors highlight the gravity of AI hallucinations, especially when they reinforce harmful stereotypes.
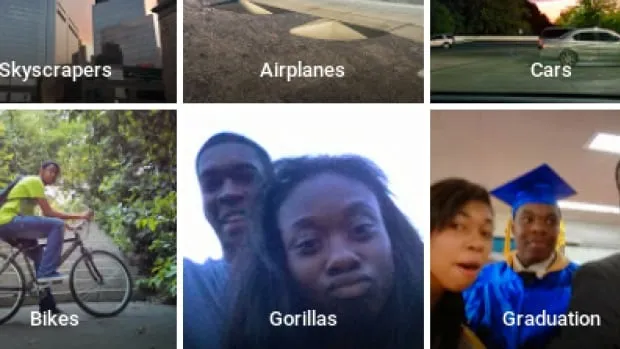
Why Are AI Hallucinations a Problem?
User Harm
AI hallucinations can lead to personal harm if users rely on incorrect information for important decisions. Misguided medical advice or inaccurate financial information are just two examples where the consequences could be severe.
Loss of Trust
When AI systems frequently hallucinate, it erodes public trust in the technology. Trust is crucial for widespread adoption and acceptance, making it essential to address the causes of hallucinations proactively.
Spreading of misinformation
The propagation of misinformation is perhaps one of the most far-reaching consequences of AI hallucinations. In an era where fake news can have global repercussions, ensuring AI-generated content is accurate and reliable is imperative to maintaining an informed society.
How to Prevent AI Hallucinations?
Use Tools that write Hallucination-Free Content
Tools designed to create hallucination-free content can help maintain the integrity of AI outputs. One such AI tool is LongShot AI. LongShot AI employs various techniques to filter and verify generated content, ensuring it aligns with factual data.
Use Diverse & Representative Training Data
To combat biases and ensure a wide range of scenarios are covered, it's crucial that training datasets are diverse and representative of real-world diversity. This helps the AI to learn a broader spectrum of patterns and reduces the risk of hallucinations.
Ground the model with relevant data
Grounding an AI model involves anchoring its outputs to reliable, fact-based information. By doing so, the risk of hallucinations is minimized as the model has a factual baseline to reference.
Always verify and fact-check
Implementing a verification step, whether through automated cross-referencing with trusted databases or human oversight, helps catch and correct hallucinations before they reach the end user. Using LongShot AI's fact-check feature can be another good idea to detect all the claims and check for their accuracy in your content.
Conclusion
AI hallucinations, while metaphorically named, present real challenges in developing reliable and trustworthy AI systems. By understanding their causes and implementing strategies to prevent them, developers can improve AI accuracy and user trust. As AI continues to integrate into our lives, addressing the issue of hallucinations becomes not just a technical challenge but a societal imperative.